AI in Futures Trading: Enhancing Forecasting and Risk Management
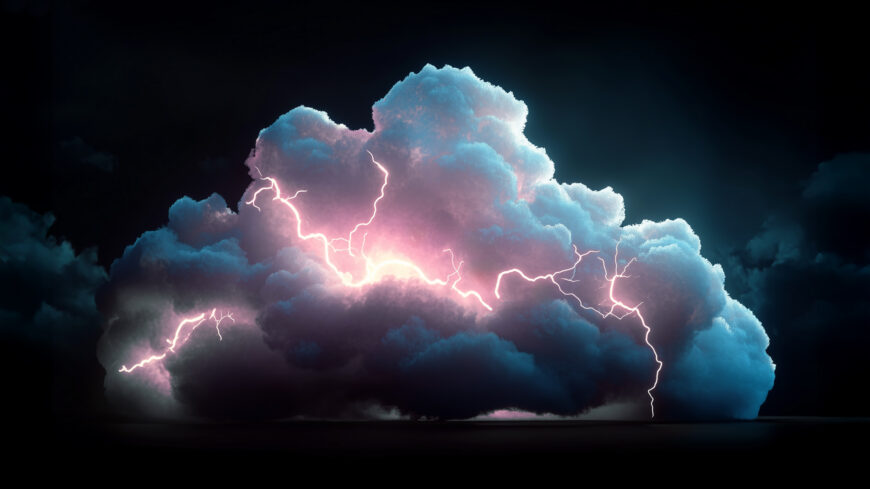
Financial institutions are rapidly integrating artificial intelligence into futures trading to gain a competitive edge. In fact, as of 2023, 99% of financial services firms reported deploying AI in some capacity. In futures markets, large data volumes, high leverage, and volatility demand advanced tools for better forecasting and tighter risk control.
This article examines how AI-driven solutions are improving data analysis and forecasting accuracy in futures trading, and how they strengthen risk management strategies, with examples from leading exchanges and banks.
AI-driven data analysis and forecasting in futures markets
AI techniques, especially machine learning (ML) and deep learning, have dramatically enhanced data analysis capabilities for futures trading. Machine learning models can sift through massive streams of market data far faster than any human analyst, flagging subtle patterns and early signals that might otherwise go unnoticed. For example, deep learning algorithms (neural networks) are used by major trading firms to recognize complex price patterns and trends, leading to more accurate predictions of futures price movements. In practice, banks and trading firms are increasingly relying on deep learning to refine their strategies and improve forecasting accuracy. By training on decades of historical futures prices and even real-time order book data, these models can forecast short-term and long-term market directions with growing precision.
One clear indicator of AI’s impact is its adoption by top quantitative trading firms. Hedge funds like Two Sigma use machine learning to forecast market trends, which helps them refine trading strategies and optimize portfolios. Such firms report that AI-driven predictive analytics gives them an edge in anticipating price movements across equity index futures, commodity futures, and other derivatives. In some studies, advanced AI models have outperformed traditional statistical methods by a significant margin—for instance, one hybrid AI approach improved prediction accuracy by 15.6% compared to older techniques. These gains in accuracy directly translate to better timing on trade entries and exits in the futures market.
AI is also broadening the scope of data that traders can analyze for forecasting. Natural language processing (NLP) models and even generative AI are now mining unstructured data, such as news feeds, earnings reports, and social media sentiment, and feeding insights into trading models. This real-time analysis of news and sentiment can improve the predictive power of trading strategies by quickly incorporating external events.
For example, an AI system might parse central bank announcements or geopolitical news and adjust currency futures forecasts within seconds, far faster than manual analysis. Additionally, AI enables the use of alternative data for price prediction. In the commodity futures arena, researchers have used satellite and aerial imagery of farmland alongside weather data to forecast crop yields and their impact on futures prices. Such an approach can give grain traders early insight into supply conditions—an AI model analyzing wheat field imagery was able to generate predictive trading signals for wheat futures based on crop health and cloud cover patterns. By integrating these unconventional data sources with market data, AI-driven analysis provides a richer, more informed basis for forecasting than was previously possible.
Overall, AI enhances forecasting in futures markets by combining speed, breadth of data, and pattern recognition. It can continuously learn from new market information, adapting its predictions as conditions change. The result is often more accurate and timely forecasts, which help traders and portfolio managers make data-backed decisions. In a field where pricing can hinge on split-second information, AI’s contribution to forecasting accuracy is proving invaluable for futures market participants.
AI-powered risk management in futures trading
Risk management is a core concern in futures trading, given the high leverage and rapid price swings involved. AI-driven systems are now playing an essential role in enhancing how firms monitor and mitigate risk. In a recent industry survey, 68% of financial services firms ranked AI-driven risk management and compliance initiatives as a top strategic priority, underscoring the industry’s focus on using AI to strengthen risk controls. Institutions like exchanges, clearing houses, and banks are deploying AI to achieve more proactive and precise risk management in several ways.
1. Real-time anomaly detection and market surveillance
AI algorithms excel at identifying anomalies or suspicious patterns in trading data that could indicate errors, fraud, or emerging risks. Deep learning models can analyze market data and client behavior to flag unusual trading patterns, detect insider trading, or prevent market manipulation.
For example, an AI-driven surveillance system might catch an irregular sequence of trades in stock index futures that hints at spoofing or algorithmic abuse, alerting risk managers immediately. JPMorgan Chase has noted that AI models scanning vast data sets are able to detect fraudulent activities and assess credit risks with greater speed, helping ensure compliance and security. By catching problems early—whether a rogue algorithm or a counterparty’s deteriorating position—AI gives risk teams more reaction time to avert losses.
2. Predictive risk analytics and early warnings
Beyond catching outright anomalies, AI is used to continuously evaluate risk exposure under changing market conditions. AI-powered systems provide early warning signals of potential market stress by monitoring a multitude of indicators in real time. In highly volatile futures markets (e.g. energy or financial futures), these systems can correlate signals from market volatility indices, trading volume surges, options order flow, and even macroeconomic indicators to warn of brewing turbulence. This kind of scenario analysis capability—described by industry leaders as like having “the risk manager’s assistant” scanning thousands of data points—gives traders and risk officers a critical edge. They can simulate “what-if” scenarios faster and more frequently, using AI to model how a portfolio might behave if, say, oil prices drop 10% overnight or if stock futures hit a limit-down scenario. The AI not only crunches the numbers faster; it often spots non-obvious correlations (for example, between an overseas market indicator and a domestic futures contract) that human analysts might miss, thereby improving the reliability of risk forecasts.
3. Accelerating complex risk calculations
Another area where AI improves risk management is in speeding up computationally intensive risk calculations. A notable example comes from Nasdaq, which integrated machine learning into its clearing and risk technology. Nasdaq’s AI-enhanced platform can perform valuation adjustments and risk metrics up to 100 times faster, enabling banks to update their risk positions almost in real time. This is critical for futures and derivatives portfolios where metrics like Value at Risk (VaR) or margin requirements need continuous recalculation as market conditions change. By vastly accelerating these calculations, AI systems allow risk managers to make timely adjustments (such as calling for additional margin or unwinding positions) to stay ahead of market volatility. The improved efficiency not only reduces operational costs but also ensures that even mid-sized firms can run sophisticated risk models without relying on external parties. In essence, AI is democratizing advanced risk analytics, making them accessible and fast for a wider range of market participants.
4. Optimizing hedging and portfolio risk
AI techniques are also enhancing how firms hedge and allocate capital against risk. Advanced ML models can recommend optimal hedging strategies by evaluating countless market scenarios. In experimental settings, reinforcement learning algorithms have been used to derive optimal hedging strategies for options, learning to maximize risk-adjusted returns under real-world constraints. While such use of AI for “deep hedging” is still emerging, more established applications exist in day-to-day portfolio risk management.
For example, AI-driven risk platforms at large banks dynamically rebalance futures positions and other derivatives in a portfolio to maintain target risk levels. They continuously quantify risk exposures and project potential losses if market factors move adversely. This helps risk managers ensure that the firm’s futures positions remain within acceptable limits and that any concentration risks or liquidity risks are flagged early.
Through these applications, AI is raising the effectiveness of risk management in futures trading. It enables earlier detection of problems, more accurate risk forecasting, and faster response times. A human risk team might review exposures once a day, but an AI can monitor them 24/7 and stress-test a portfolio against hundreds of scenarios in minutes. The end result is a more resilient trading operation. Firms like CME Group and other exchanges are leveraging AI to augment their risk management frameworks, from clearinghouse margin models to market surveillance (even as regulators like the CFTC emphasize that proper oversight and governance of AI models is essential in this highly regulated domain. The key for organizations is to combine these AI tools with human expertise—using AI’s output to inform decisions, while risk officers set policies and intervene when needed.
Conclusion
At Devexperts, we work closely with brokers, banks, and trading firms to develop technology solutions that meet the demands of modern futures markets. The AI-driven capabilities described in this article—ranging from real-time data analytics to risk monitoring and predictive modeling—are no longer optional for firms aiming to stay competitive. They’re becoming core components of resilient, high-performance trading infrastructure.
If you’re looking to incorporate similar AI-powered tools into your futures trading platform, or facing specific operational and risk management challenges, we’re here to help.
Get in touch with our team to explore how Devexperts can support your next step forward.